Scientific publications play a pivotal role in advancing our understanding of the world, and their credibility hinges on the accurate interpretation and reporting of data. Within this context, the term “trend” holds significant weight, often serving as a key descriptor of observed patterns. The inappropriate use of this term for non-significant data, however, poses a serious concern in scientific discourse. Here we discuss the complexities surrounding the identification and communication of trends, emphasizing the importance of precision in statistical interpretation and reporting. By exploring the consequences of misusing the term “trend” in the absence of statistical significance, we underscore the critical need for clarity and accuracy in scientific publications. A nuanced understanding of statistical concepts and a commitment to best practices are essential for maintaining the integrity and reliability of scientific research.
Also read:
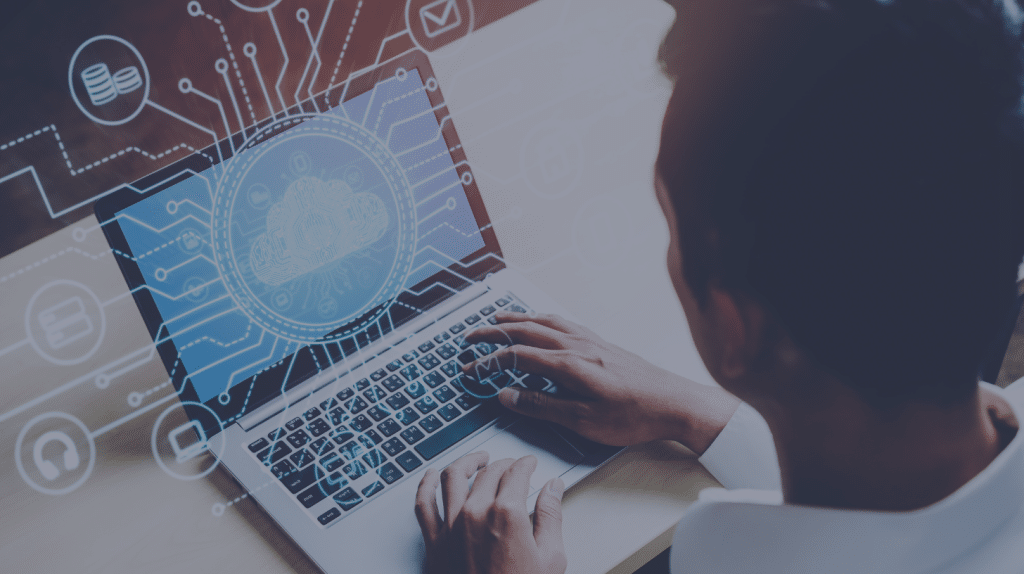
The Nature of Trends
In scientific research, a trend is commonly understood as a discernible pattern or directionality in data over a certain period. This extends beyond mere visual observations, however, and requires rigorous statistical scrutiny to validate its significance. Statistical trends are characterized by a consistent pattern that goes beyond random variation, highlighting the importance of distinguishing between meaningful trends and fluctuations due to chance.
To differentiate a genuine trend from random variation, researchers rely on statistical tests that provide a measure of confidence in the observed patterns. Significance levels, often represented by p-values, are crucially involved in this determination. A trend is typically considered statistically significant when the probability of observing the observed pattern by random chance is low, typically below a predetermined threshold (α, acceptable type I error; commonly 0.05). If p≤0.05, the null hypothesis (the assumption that no difference exists) is rejected. A p>0.05 is considered to indicate that the null hypothesis cannot be rejected. That is, there are 2 possible outcomes. There is no p-value that represents a trend. Yet, in the literature, “trend” is often applied to mean an almost significant difference – as if the p-value is moving towards the cutoff value and just did not quite make it.
For example:
A trend toward improvement was observed for higher doses of the drug (p<0.06).
This is a misinterpretation of the results as p<0.06 does not meet the predetermined value, period. Simply stated, higher doses of the drug had no improving effects.
Understanding and applying these statistical principles are paramount to accurately characterizing trends and avoiding the mislabeling of non-significant patterns.
Consequences of Misusing “Trend” for Non-Significant Data
Misapplication of the term “trend” to non-significant data can have far-reaching consequences for the scientific community and beyond. By presenting non-significant findings as trends, researchers risk misleading readers, perpetuating inaccuracies, and contributing to a distorted understanding of the phenomena under investigation. This miscommunication has the potential to influence subsequent research directions, misguide policy decisions, and erode public trust in scientific findings.
The credibility of scientific publications is at stake when trends are inaccurately characterized. Fellow researchers, policymakers, and the public rely on the accuracy of published data to make informed decisions. Misuse of the term “trend” undermines the integrity of scientific literature, potentially hindering the progress of knowledge in a given field. The literature contains multiple examples of how these types of miscommunication have influenced subsequent studies and policies in various fields.
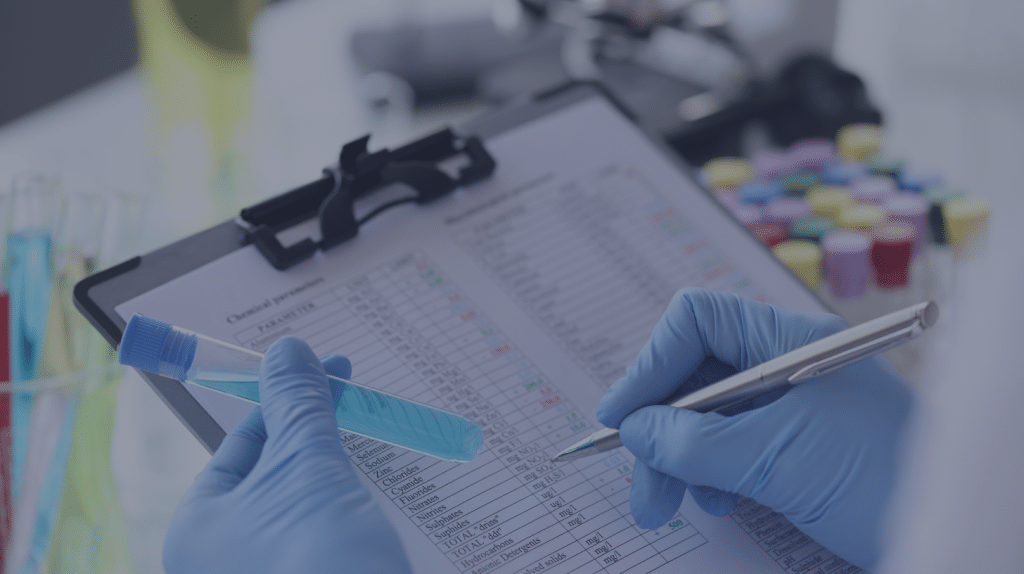
Common Pitfalls in Identifying Trends
Several common pitfalls contribute to the mislabeling of non-significant data as trends in scientific publications. One prevalent issue is the overemphasis on visual patterns without robust statistical validation. Researchers may be tempted to interpret apparent trends based on visual inspection alone, neglecting the need for statistical tests to confirm the significance of these patterns.
Misinterpretation of p-values and statistical significance further compounds the problem. In some cases, researchers may mistakenly believe that a small p-value is synonymous with a substantial or meaningful effect, overlooking the importance of effect size and practical significance. Additionally, inadequate consideration of sample size and statistical power can lead to false interpretations of trends, as small sample sizes may fail to detect genuine effects.
Addressing these pitfalls requires a commitment to sound statistical practices, emphasizing the importance of comprehensive analysis and interpretation.
Best Practices to Avoid Misuse of “Trend” in Non-Significant Data
To mitigate the risk of misusing the term “trend” in non-significant data, researchers and authors must adhere to best practices in statistical analysis and reporting. Rigorous statistical analysis and interpretation are essential, involving the use of appropriate statistical tests to determine the significance of observed patterns. Clear reporting of p-values and confidence intervals provides readers with the necessary information to assess the robustness of findings.
Transparent communication of limitations and uncertainties is equally crucial. Authors should explicitly acknowledge the presence of findings in which the p-value approaches the cutoff value (e.g., 0.05) but does not reach it and discuss potential reasons for their occurrence (e.g., study was underpowered, variability, etc.), suggesting further studies designed to address the issues more directly. By openly acknowledging the limitations of the study, researchers contribute to a more accurate and nuanced scientific discourse.
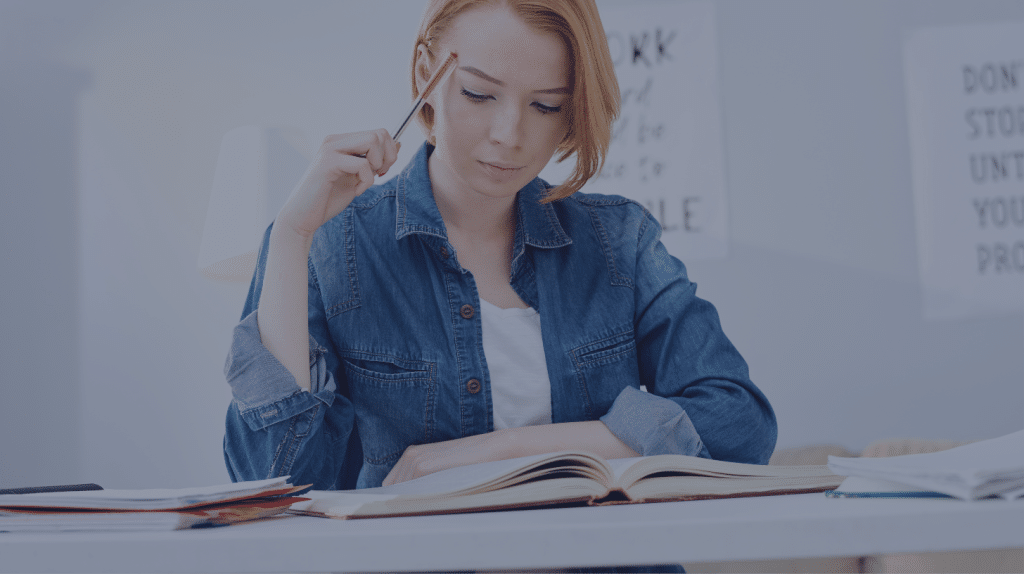
Case Studies
To illustrate the consequences of misusing the term “trend” for non-significant data, we examine several case studies drawn from scientific literature:
- Case Study 1: Climate Change Indicators
- In a study assessing climate change indicators, researchers observed a slight upward trend in temperature data over a short timeframe. Despite the trend not reaching statistical significance, the term “warming trend” was used in the publication’s summary, potentially misleading readers and policymakers. This misinterpretation could contribute to exaggerated perceptions of the immediacy and severity of climate change.
- In a study assessing climate change indicators, researchers observed a slight upward trend in temperature data over a short timeframe. Despite the trend not reaching statistical significance, the term “warming trend” was used in the publication’s summary, potentially misleading readers and policymakers. This misinterpretation could contribute to exaggerated perceptions of the immediacy and severity of climate change.
- Case Study 2: Drug Efficacy in Small-Scale Trials
- A pharmaceutical study investigating a new drug reported a noticeable improvement in a particular symptom among participants. Due to the small sample size, however, the observed trend did not achieve statistical significance. Nonetheless, the term “positive trend” was used in the conclusions, possibly leading to unwarranted enthusiasm about the drug’s efficacy. This could have implications for subsequent research directions and patient expectations.
- A pharmaceutical study investigating a new drug reported a noticeable improvement in a particular symptom among participants. Due to the small sample size, however, the observed trend did not achieve statistical significance. Nonetheless, the term “positive trend” was used in the conclusions, possibly leading to unwarranted enthusiasm about the drug’s efficacy. This could have implications for subsequent research directions and patient expectations.
- Case Study 3: Educational Intervention Outcomes
- A study evaluating the effectiveness of an educational intervention identified a positive trend in test scores among participants. Although the trend did not reach statistical significance and the researchers cautiously stated the findings in the discussion section, media coverage focused on the perceived positive trend, potentially misinforming the public about the intervention’s impact.
In each case, the misuse of the term “trend” for non-significant data had implications for the broader understanding of the phenomena being studied. These examples underscore the importance of precise and transparent reporting, as well as the need for researchers to exercise caution in interpreting and communicating trends that lack statistical significance.
Conclusion
In conclusion, the term “trend” in scientific publications holds immense weight, and its misuse for non-significant data can have far-reaching consequences. As demonstrated through case studies and analysis, the inadvertent mislabeling of non-significant findings as trends can mislead readers, influence subsequent research, and erode the credibility of scientific literature.
To safeguard the integrity of scientific discourse, researchers must commit to rigorous statistical practices, transparent reporting, and clear communication of limitations. By avoiding common pitfalls and adhering to best practices, researchers can ensure that trends identified in data are not only visually apparent but also statistically robust. It is imperative that researchers, editors, and reviewers collectively prioritize accuracy and precision in reporting to maintain the trustworthiness of scientific knowledge.
AI Disclaimer: This article was generated with the assistance of ChatGPT, an AI language model, to enhance its content. The AI-generated content was thoroughly reviewed, rewritten, and edited to ensure accuracy and coherence. The authors take full responsibility for this article.